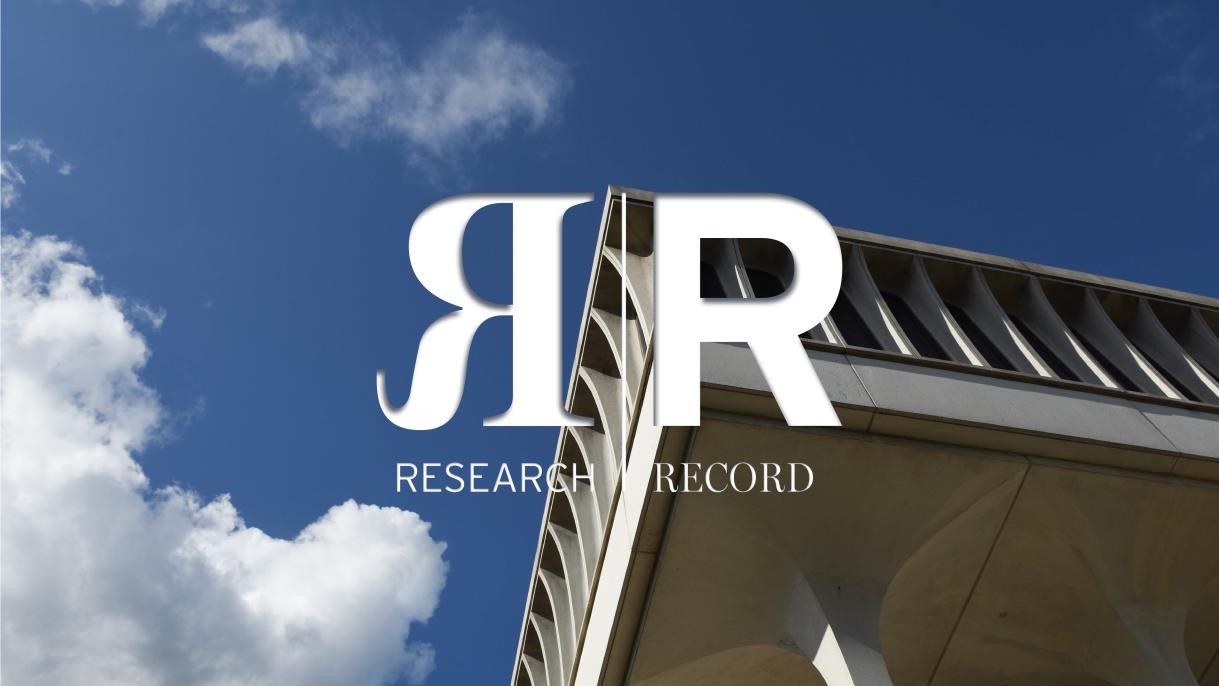
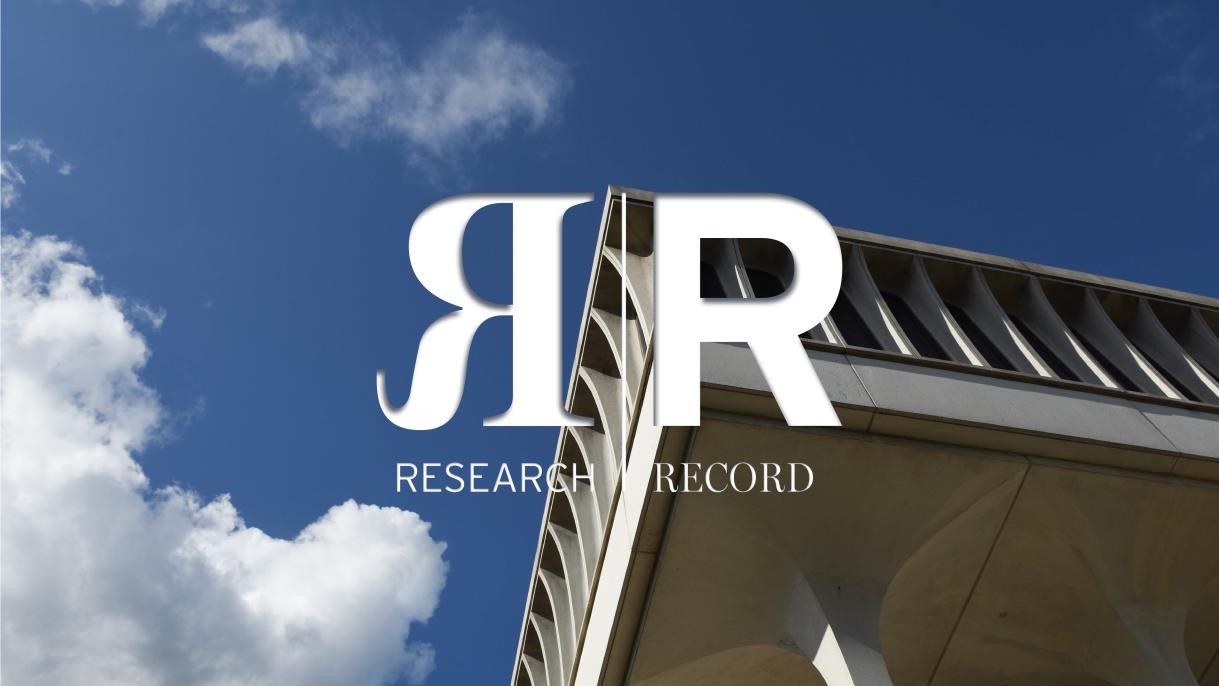
Research Record: Examining the Origins of Life Predictability
Princeton SPIA’s Research Record series highlights the vast scholarly achievements of our faculty members, whose expertise extends beyond the classroom and into everyday life.
If you’d like your work considered for future editions of Research Record, click here and select “research project.”
The Details
Authors: Kathryn Edin (Princeton University), Matthew Salganik (Princeton University),
Timothy Nelson (Princeton University), Sarah Pachman (Princeton University), Rachel
Brown-Weinstock (Princeton University), Vicki Yang (Princeton University), Ian Lundberg
(Cornell University), Susan Clampet-Lundquist (St. Joseph’s University)
Title: The origins of unpredictability in life outcome prediction tasks
Journal: Proceedings of the National Academy of Sciences of the United States of America
The Big Picture
The research team sought to understand the origins of unpredictability in life outcome prediction tasks. For example, why does a child who shows signs of doing well in school sometimes drop out while others excel?
“People’s lives are remarkably hard to predict,” the authors said. “You might know a lot about a child through elementary school, yet still struggle to predict their performance in high school even with high-quality machine learning methods. It would be terrific if we could predict which children would struggle academically in high school. We might be able to direct extra support to them. Yet when predicting life outcomes we must also beware that unpredictability may be large.”
The Findings
The team identified two sources of unpredictability.
The first is that two people who exhibit similar characteristics at one stage of their lives may have very different outcomes in the future.
“You can think of two kids with equally good grades in elementary school, who have dramatically different outcomes in high school,” they said. “To the degree that two people who look the same have very different outcomes, there are limits to how well one can predict even with the best machine learning. We call this an irreducible error.”
Second, the research team identified that there might be patterns that statistical and data science methods learn inaccurately — or a learning error.
“We think that irreducible and learning errors are likely to be large in many life outcome prediction tasks,” the coauthors said. “Most broadly, in social science, we often focus on patterns of predictability — how one variable consistently predicts another. But sometimes a big part of the story is unpredictability — how outcomes turn out differently from what might be expected, especially over the life course.”
The Implications
“Prediction could improve policy, but one should be aware of limits to prediction,” the coauthors said. “Our project may help policymakers to think about the degree of predictability that can be expected in a given task. We think this might help them think more carefully about the usefulness or limits of prediction in policy. We also hope our study spurs new research and policy discussion about unpredictability in human lives, which we think is an intrinsically valuable area of future work.”